Note
Go to the end to download the full example code. or to run this example in your browser via Binder
Tidal Elevation Prediction using LPG Discretization#
This example demonstrates how to use the model with LPG discretization to predict tidal elevation in a specified area of interest.
Warning
The model employed is an older FES tidal-atlas model due to its significantly smaller size compared to newer models. Do not use it for real applications. You can download the model from the AVISO website.
First, we import the required modules.
from __future__ import annotations
import os
import pathlib
import cartopy.crs
import matplotlib.pyplot
import numpy
import pyfes
First we create an environment variable to store the path to the model file. The configuration file is fully documented in the documentation.
Note
The content of the configuration file is viewable in the GitHub repository.
os.environ['DATASET_DIR'] = str(pathlib.Path().absolute().parent / 'tests' /
'python' / 'dataset')
handlers: dict[str, pyfes.core.AbstractTidalModelComplex128
| pyfes.core.AbstractTidalModelComplex64]
handlers = pyfes.load_config(pathlib.Path().absolute() / 'fes_lpg.yml')
handlers
is a dictionary that contains the handlers to the ocean and
radial tide models.
print(handlers)
{'radial': <pyfes.core.tidal_model.CartesianComplex64 object at 0x7f6bf7fa7db0>, 'tide': <pyfes.core.tidal_model.LGP2Complex64 object at 0x7f6bf7f863b0>}
We can now create a grid to calculate the geocentric ocean tide around the french coast.
lons = numpy.arange(-5, 40, 0.25)
lats = numpy.arange(10, 55, 0.25)
lons, lats = numpy.meshgrid(lons, lats)
shape = lons.shape
dates = numpy.full(shape, 'now', dtype='datetime64[us]')
We can now calculate the ocean tide and the radial tide.
tide, lp, lpg_flag = pyfes.evaluate_tide(handlers['tide'],
dates.ravel(),
lons.ravel(),
lats.ravel(),
num_threads=0)
load, load_lp, _ = pyfes.evaluate_tide(handlers['radial'],
dates.ravel(),
lons.ravel(),
lats.ravel(),
num_threads=0)
We can now calculate the geocentric ocean tide (as seen by a satellite).
geo_tide = tide + lp + load
geo_tide = geo_tide.reshape(lons.shape)
Mask the land values.
geo_tide = numpy.ma.masked_where(numpy.isnan(geo_tide), geo_tide)
We can now plot the result.
fig = matplotlib.pyplot.figure(figsize=(15, 10))
fig.suptitle(f'Tide and Interpolation Quality Flag on {dates[0, 0]}',
fontsize=16)
# Plot the geocentric ocean tide
ax1 = fig.add_subplot(1, 2, 1, projection=cartopy.crs.PlateCarree())
ax1.set_extent([-5.0, 10.0, 40.0, 55.0], crs=cartopy.crs.PlateCarree())
ax1.coastlines()
ax1.set_title('Geocentric Ocean Tide')
ax1.set_xlabel('Longitude')
ax1.set_ylabel('Latitude')
mesh1 = ax1.pcolormesh(lons,
lats,
geo_tide,
cmap='jet',
transform=cartopy.crs.PlateCarree())
colorbar1 = fig.colorbar(mesh1, ax=ax1, orientation='horizontal', pad=0.05)
colorbar1.set_label('Geocentric ocean tide (cm)')
# Plot the interpolation quality flag
ax2 = fig.add_subplot(1, 2, 2, projection=cartopy.crs.PlateCarree())
ax2.set_extent([-5.0, 10.0, 40.0, 55.0], crs=cartopy.crs.PlateCarree())
ax2.coastlines()
ax2.set_title('Interpolation Quality Flag')
ax2.set_xlabel('Longitude')
ax2.set_ylabel('Latitude')
mesh2 = ax2.pcolormesh(lons,
lats,
lpg_flag.reshape(lons.shape),
cmap='Dark2_r',
transform=cartopy.crs.PlateCarree(),
vmin=0,
vmax=4)
colorbar2 = fig.colorbar(mesh2, ax=ax2, orientation='horizontal', pad=0.05)
colorbar2.set_label('Interpolation Quality Flag')
fig.tight_layout()
matplotlib.pyplot.show()
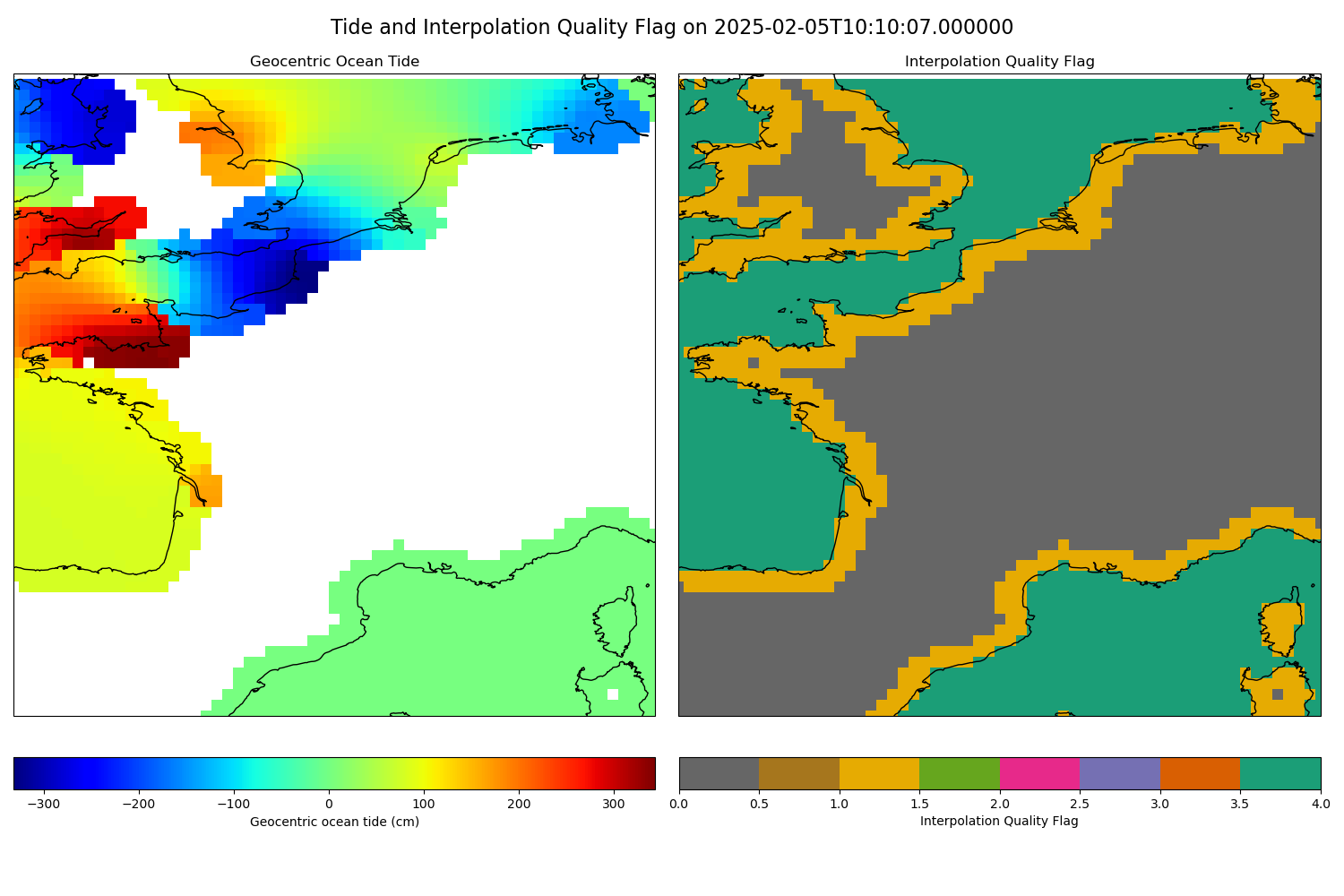
Total running time of the script: (0 minutes 3.842 seconds)